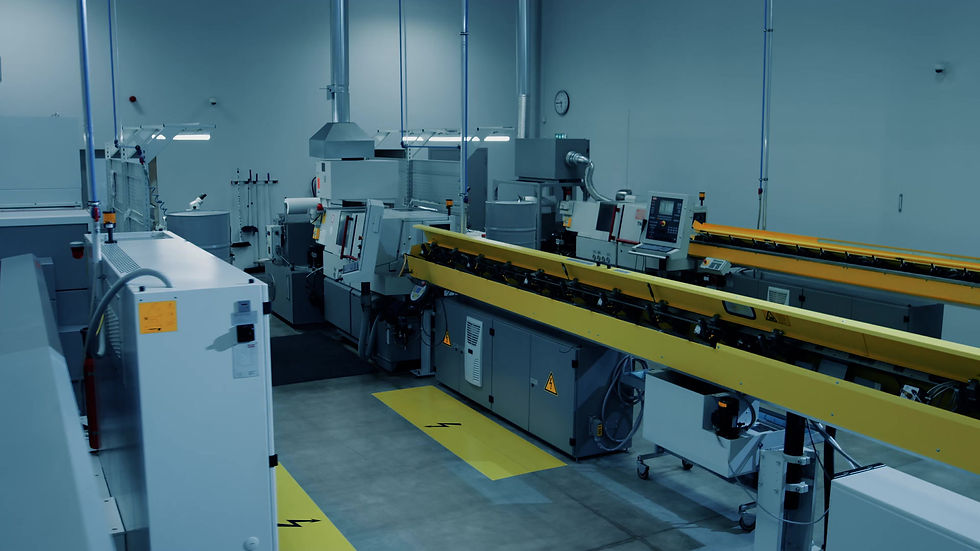
LEAP
TECHNOLOGIES
Bridging the Gap Between Conventional Mechatronics Engineering and Data Science
OUR CLIENTS
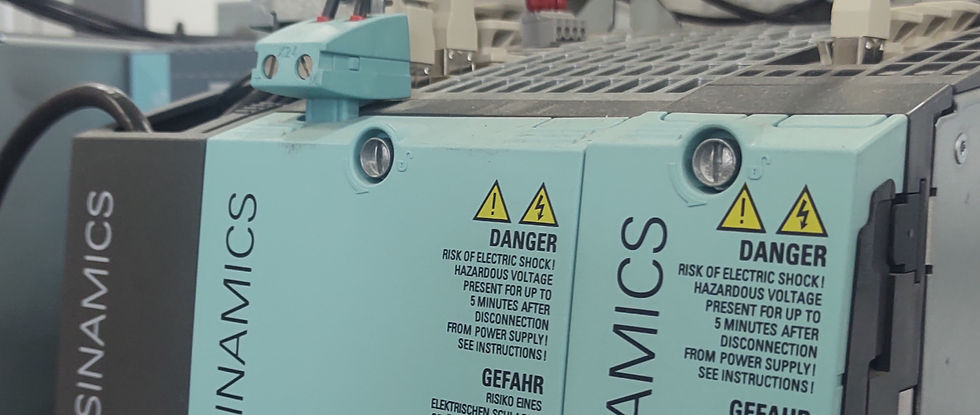
ABOUT US
As an official spin-off originating from the controls engineering group of Ghent University, we continuously seek for synergies between novel data-driven and conventional solutions to optimize the performances of mechatronic applications. Together with various industrial partners, we have successfully demonstrated the power of using data-driven solutions for enhancing the control, reliability and intelligence of machines. We endeavor to start each project from a hands-on approach, while always keeping in touch with the latest trends.
OUR ACTIVITIES
If you have specific questions or research challenges related to data-analytics, control, modeling or IoT, do not hesitate to contact us. We endeavor to align our can-do attitude with a pragmatic and down-to-earth way of approaching challenges so that we can optimally work towards the desired outcome of the assignment.

ADVANCED AUTOMATION
MODELING
& CONTROL
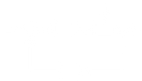
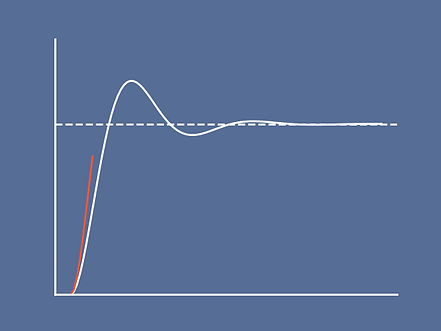
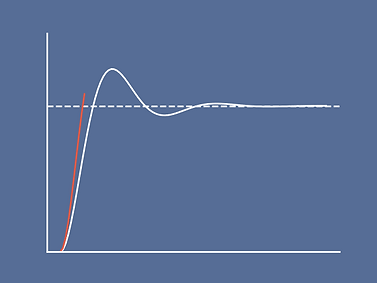
Using the right system modeling techniques, we strive to upgrade your system control unit to the next level. By having a strong foundation and experience in adaptive control algorithms we enable robust automatization strategies that are capable of handling variable environments.

VIRTUAL SENSING
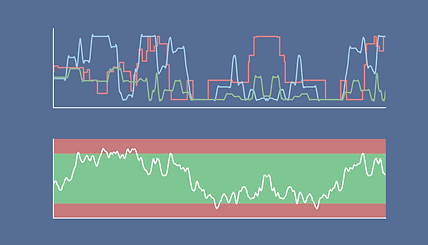
Through the creation of virtual sensors, we can monitor process parameters that lack dedicated sensors or would otherwise incur substantial expenses. By employing data-driven and physics-based relations, we construct estimation models capable of overseeing critical system properties, offering real-time adaptability when integrated into the control loop.
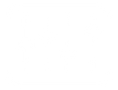
SETTING AUTOMATION
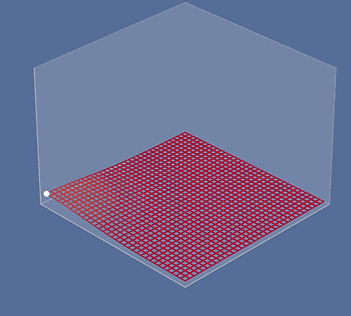
To mitigate the influence of operator expertise and intuition on machine performance, historical data can be used to unveil the connections between system configurations, process parameters, and operational efficiency. By incorporating these relations into the controller, we can automate setpoint recommendations, thereby reducing the reliance on operator skills.
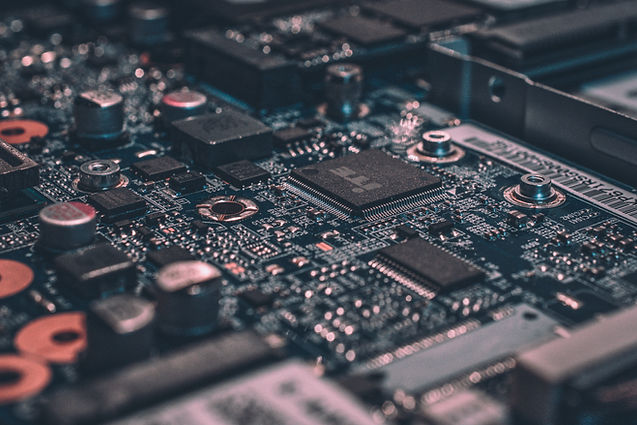
DATA SCIENCE
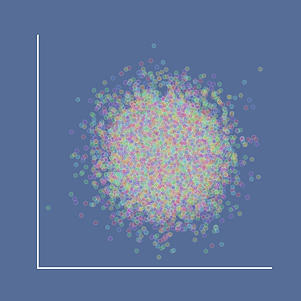
Analyzing a large volume of data extracted from control units, such as PLCs, allows us to discover hidden correlations and relationships within the machine's data. This empowers us to deliver statistically proven feedback to the engineering team.
DATA MINING

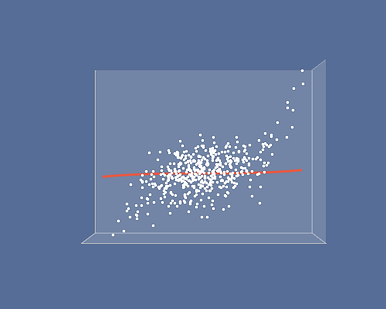
Our expertise extends from fundamental statistics to advanced machine learning techniques. This enables us to create models for accurate predictions of the system's behavioral performance, paving the way for rapid interventions and operational optimization of the machines.
DATA ANALYTICS
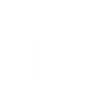
We provide custom computer vision solutions that enable the quantification of process quality and output. Moreover, when being integrated into the control loop, computer vision can serve as robust feedback for real-time adjustments.
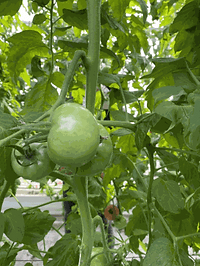
COMPUTER VISION


SPIN-OFF
To keep in touch with the novel trends in datascience, system identification and control, we maintain, as an official spin-off, a strong collaboration with our alma mater, and more specifically the dynamics research team led by prof. Guillaume Crevecoeur. This way we endeavor to form a link between the recent advances in academia and the industry. Currently, we work together on the following projects:
The hybrid modeling framework provides a toolchain for learning system models of mechatronic applications by combining both physics-inspired and data-driven components. The resulting hybrid models offer a means for learning reliable system models that can accurately predict the behavior of complex machines. Moreover, by extracting the data-driven relations after convergence, new insights about initially unknown phenomena (e.g. friction, disturbances …) can be learned from the model.

HYBRID MODELLING
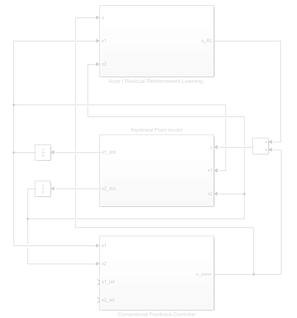
RESIDUAL REINFORCEMENT LEARNING
Residual Reinforcement Learning (RRL), is a method developed at Ghent University that combines the robustness of a conventional controller with the flexibility of a data-driven controller. RRL overlays the conventional controller with a data-driven controller that is allowed to find small adaptations necessary to reattain optimality in new conditions, while the conventional controller provides the bulk of the control input and ensures robustness at all times.